Why Life Sciences Companies Need More than ChatGPT for Analytics
.avif)
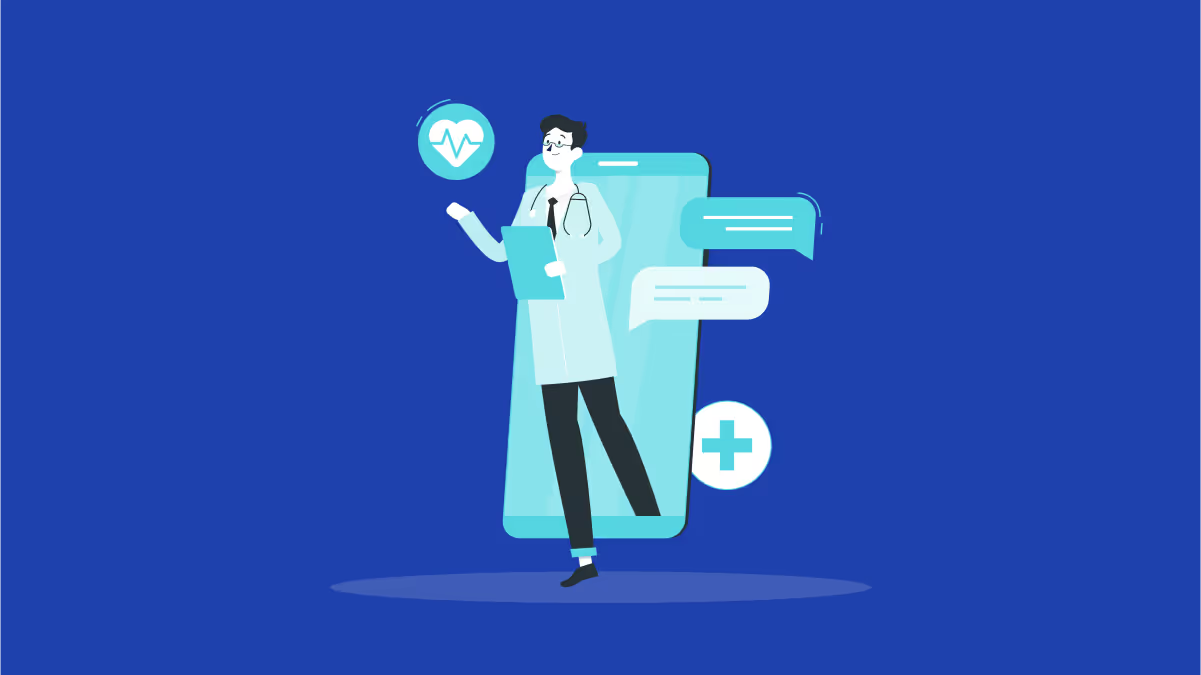
Awareness, Realization of Potential and Trust in Conversational AI
Society has had a complex relationship with artificial intelligence (AI), anxiously anticipating what this technology can do to simplify work and enhance lives – but at the same time, viewing it with a degree of skepticism and, perhaps, distrust. But when ChatGPT burst onto the scene in November 2022, it sparked discussions about AI from tables in the kitchen to the boardroom about what’s possible with conversational AI systems – and how ChatGPT proves they can work. ChatGPT’s release will likely mark a turning point toward widespread awareness, trust, and a greater understanding of AI’s potential.
What ChatGPT is and Does – and What It Doesn’t Do Well
ChatGPT is a generative conversational AI system based on GPT-3.5 Large Language Model (LLM). It uses deep learning to process large amounts of unstructured text data. The model can understand language and identify relationships, enabling it to answer even complex questions. It’s easy to believe that ChatGPT is creating original outputs. But the truth is, it’s stitching together answers based on its training datasets. The key to the algorithm is its ability to predict the next word or sentence contextually and then keep building on top of it.
ChatGPT’s training data was current through 2021. However, it uses interactions with users to continue to learn, using inputs and user feedback to improve accuracy (a technique called Reinforcement Learning). The downside of this feature for users is that whatever you share, ChatGPT will use, so users need to be cautious about sharing sensitive or proprietary information.
Since its launch as a free research preview, curiosity-seekers have discovered a wide range of ways to use ChatGPT. For example, the tool can compose music or poems, write software code, and solve math problems. It’s also inspiring company leaders to explore how conversational AI could help transform their businesses.
Life Sciences Analytics Challenges with Large Structured Data Systems
Life sciences companies that explore analytics and building a conversational AI system from the ground up quickly find that there are major hurdles to overcome, including:
- Data volume: Life sciences analyses can use data from petabytes of internal and external sources. Organizations need an effective way to manage, categorize, process, and analyze ever-growing volumes of historical and dynamic data.
- Data silos: Systems that hold valuable insights may have their own data repositories. Life sciences companies need a single source of truth that makes all data available for analysis.
- Data integration: Life sciences companies can be overwhelmed by the number of data sources in different systems that must be integrated with the analytics tool.
- Data relationships: Companies also need a way to recognize the relationships between data and data sets so they can understand which data is relevant to the analyses they need to perform.
- Data quality: An AI model will only provide high-quality, accurate insights if it’s trained with and uses high-quality, accurate data. Data quality monitoring is critical for a system that provides value.
- Data security: Life sciences data includes protected information, intellectual property (IP), and sales and marketing data. It must be protected from hackers seeking to monetize it or competitors that could use it to their advantage.
- Data governance: Organizations must also establish policies for storing, managing and using data and monitoring compliance with those policies.
Importance and Significance of Domain-Specificity
While it’s possible to use a model without a specific domain focus, AI works best for a domain when it’s specifically trained for it. In life sciences, for example, it takes months and significant investment to train an AI system for life sciences data to deliver the accurate insights required. Moreover, life sciences data is always changing, so training a model once and then using human interaction to learn isn’t adequate to keep up with the most current healthcare provider, payer, patient, competitor, and internal data.
WhizAI has been trained on life sciences data sets and was designed to simplify the discovery of insights on large and complex data sets. These insights are relevant to the users and personalized to their individual preferences. The core AI components or building blocks are natural language processing and understanding so a user can converse with the system. This is not keyword based and it is not search based, it is pure natural language. Once the system understands what the users are asking for, it needs to determine what is the best way to present this information. So, we have AI models that select the right visuals. Next we provide guided analytics. The system learns the line of questioning in analytics, how the users are querying for data and applies these learnings to suggest what should be the next best question that the user could ask.
WhizAI offers life sciences enterprises automated insights such as key driver (causal) analysis, anomaly detection and predictive analysis. If prescriptions for a specific brand decrease, for example, WhizAI can compare its performance to a competitive product and drill down into regional changes or payer decisions to determine the root cause. Furthermore, WhizAI can identify patterns that show what might happen next or what to expect if you make a change.
WhizAI and ChatGPT Similarities, Differences, and Purposes
AI, even a bot that can produce a range of different types of outputs, is always built for a purpose. ChatGPT is trained with text to deliver human-like responses when a user asks a question or gives it a prompt.
WhizAI’s conversational AI for life sciences analytics, on the other hand, is trained with numbers – enterprise datasets, metrics, and life sciences and healthcare data from external sources – not limited to text. However, WhizAI also uses LLM to understand users’ intents, ask questions conversationally and infer outputs from the data, presenting them as visualizations that quickly show relationships and answer questions.
Think of it this way. ChatGPT gives you human-like answers with words. WhizAI provides analyst-like responses with storyboards, graphics, representations, and visuals. Life sciences enterprises will benefit more from a system that acts like an analyst.
.png)
The Future of AI
Adoption will grow as users discover that an LLM-based system can deliver reliable results and learn their personal preferences from interactions. Users can also expect a de facto interface for AI, standardizing how people interact with AI across multiple devices or embedded in business applications. The future of AI also includes prescriptive and preventive analyses, enabling models to not only describe the current state but also to suggest the best course of action to users. This will manifest as people in all roles of an organization having easy access to insights and building data-driven decision making into their day-to-day workflows.
Growing awareness, trust, and comfort with AI are all signals that ubiquity is possible. Of course, much work remains to achieve widespread use, but tools like ChatGPT and WhizAI demonstrate that businesses can enhance operations with artificial intelligence.
Learn more about how ChatGPT has validated the approach WhizAI adopted five years ago or request a live demo today.