4 Key Capabilities to Look for When Evaluating an AI-Powered Analytics Platform for Life Sciences Business Users
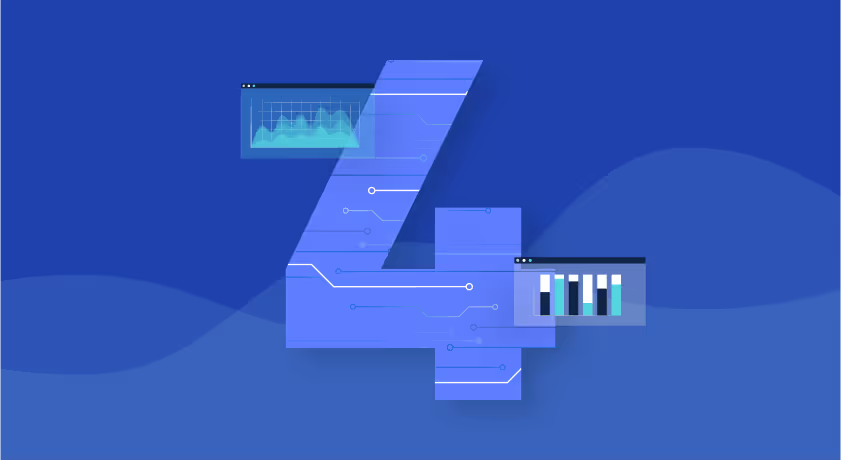
Artificial intelligence (AI) is crucial to unlocking value from life sciences data. First, it’s up to the challenge of dealing with massive – and continually growing -- data volumes. IDC estimated healthcare and life science data would increase by 270 GB in 2020 for each of the world’s 7.9 billion people. Next, AI is fast. It can perform complex tasks, such as analyzing billions of data points from multiple sources in less than a second. Additionally, AI-powered analytics can continue to learn, providing more precise, relevant responses to queries over time.
Perhaps the most beneficial aspect of AI is its ability to make decision analytics accessible to everyone. The WhizAI webinar, Life Sciences AI: Augmented Analytics Consumers with a Vertical Approach, detailed how AI enables every employee in a life sciences organization to make data-based decisions. As Chris Ilacqua, Director, Product & Design for WhizAI, pointed out, that advantage extends even “to people without data analyst in their titles.”
However, not every AI-powered analytics platform is equal in its ability to provide insights to life sciences teams. When you vet platforms for your organization, determine how they measure up in these four capability areas essential to helping your company get the desired ROI from data and augmented analytics investments.
Cognitive Insights
A life science analytics platform needs to do more than return results to a query, similar to a search engine. The platform should leverage deep learning to provide predictive analytics for forecasting and projections.
The optimal platform will also automatically surface insights and alerts based on data trends or anomalies. It will even have the capability to anticipate the next question a user will ask. Users shouldn’t have to change habits or workflows to use an augmented consumer analytics platform. The platform should adapt to users.
A Hybrid Natural Language Processing Engine
In addition to deep learning, AI-powered analytics platform for life sciences should also utilize sophisticated linguistic algorithms. Combining these technologies in a platform trained with domain-specific data is a powerful tool for life sciences teams. It understands the intent of questions, even if analytics consumers ask them differently or if some information is missing.
Ilacqua points out that deploying a conversational analytics platform designed to work across vertical markets would take months to train for life sciences. However, an AI-driven analytics platform pre-trained for the domain provides that value out of the box, delivering quick time to value.
Zero Code Environment
Suppose increasing data-based decisions throughout your organization is driving the search for an augmented consumer platform for life sciences. In that case, you need to ensure that everyone on your team can use it easily. The analytics solution shouldn’t require users to configure it to perform calculations or to use established keywords or phrases to get it to work.
The ideal self-service analytics solution will mimic human conversation. Users simply ask questions, just as if they were speaking to colleagues. The platform responds with easy-to-understand data visualizations.
Enterprise-Readiness
The top solution for life sciences companies will be purpose-built from the ground up with microservices architecture managed via Kubernetes for reliability, uptime, and scalability. It will also include out-of-the-box adapters for integrating life sciences data sources, delivering sub-second responses at any scale, and protecting data with enterprise-grade security.
Enterprises will also benefit from a solution embedded into other applications, such as Salesforce, Microsoft Teams, or Veeva, so analytics consumers can access it within their typical workflows, using the devices they usually use.
Solutions should also support multiple languages to meet the needs of global enterprises.
A Decision You Need to Make
Continuing to rely only on legacy analytics processes that can take weeks to deliver the insights users need is not an option in a dynamic industry. Grand View Research predicts the life science analytics market will grow at a 7.7% CAGR through 2030. Their analysts point out that although descriptive analytics led the market in 2021, predictive analytics adoption will grow throughout the forecast period. Life science enterprises are expanding their capabilities to provide their employees with insights that guide the best next steps.
Organizations on the cutting edge of analytics will have an advantage. Rohit Vashisht, CEO and Co-Founder of WhizAI, observes, “Our life sciences customers are steadily gaining a market edge. Because of its domain focus, WhizAI provides unparalleled speed and timely insights, creating data-driven business teams that can act quickly and efficiently. The future of analytics is automated, simple and contextual. Domain specificity gets us there.”
To see how WhizAI’s enterprise-ready AI-powered analytics platform delivers cognitive insights to the information consumers in life sciences, request a demo of our platform.