What are the Key Components of Next-Gen Augmented Analytics Tools?
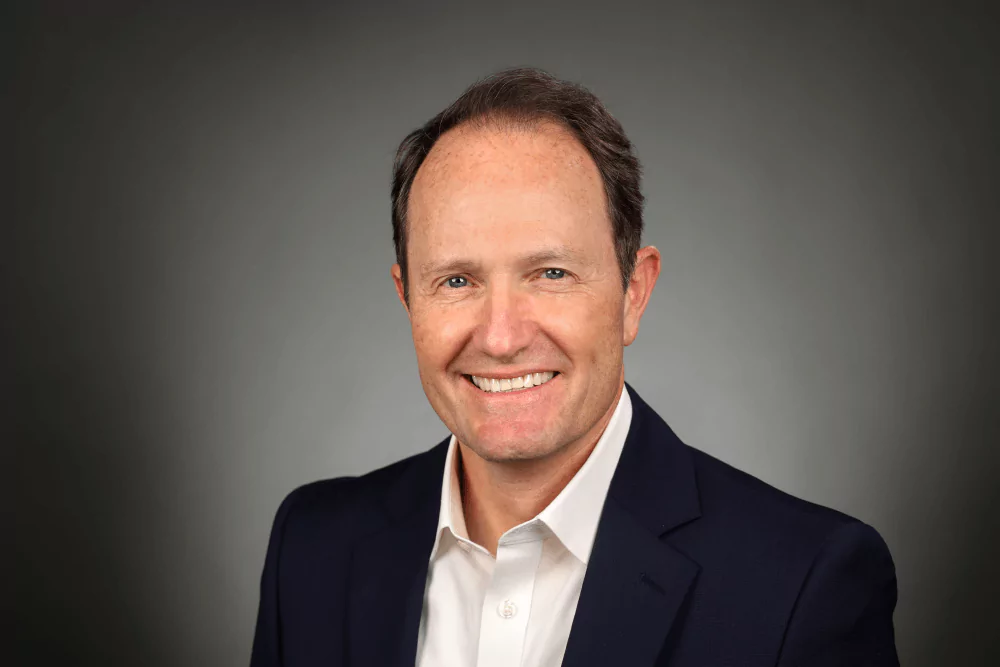

Life sciences teams can do their jobs more effectively when data-driven decision-making is a part of their workflows. With access to data insights, field sales can tailor presentations with physician-level details, and the market access team can identify hurdles to adherence. However, making data insights readily available to these life sciences professionals has been challenging. Traditional analytics and business intelligence platform require data science expertise and time to build dashboards and visualizations. With those platform, it’s impossible to quickly identify a new sales opportunity or solve an urgent issue with access to treatment. But augmented analytics tools give business users autonomy.
What Are Augmented Analytics Tools?
Augmented analytics tools use advanced technologies, such as artificial intelligence (AI), machine learning (ML), deep learning, natural language processing (NLP), and low-code/no-code capabilities, to make analytics accessible to business users. Augmented analytics makes it easier for humans to interact with data, even if they don’t have data science expertise, and to get answers to their questions and analytics insights on demand.
Features of Optimal Augmented Analytics Tools for Life Sciences
Several components and techniques work together to make data insights more accessible to life sciences teams:
- AI: Artificial intelligence (AI) enables giving an augmented analytics tool for life sciences end-to-end contextualization. Platforms trained with life sciences data provide accurate, contextual answers from day one, building user trust more quickly and encouraging adoption. Domain-specific platform also enable faster implementation, reducing implementation time from months to weeks.
- ML: Machine learning (ML) gives an augmented analytics tool the ability to rapidly search large volumes of data in a short period. WhizAI, for example, can search billions of data points in just a few seconds to answer questions based on historical data, identify patterns and anomalies, and offer the next steps. Subsets of ML, including deep learning, natural language processing (NLP) and natural language understanding (NLU), give the platform additional capabilities, including greater usability.
- Intelligent automation: An augmented analytics tool that decreases the need for human intervention in analytics also reduces the time from data acquisition to insights. With intelligent automation, a platform can facilitate key performance indicator (KPI) monitoring as well as deliver real-time insights.
- NLP: NLP enables users to interact with the augmented analytics tool conversationally. NLU allows the tool to understand both the meaning and intent of users’ questions, and natural language generation enables it to provide a contextual response that users can easily understand.
- Low-code/no-code environment : Platforms that require users to code to configure their interfaces or perform other functions are difficult, if not impossible, for all members of commercial teams or other life sciences employees to use. Businesses should be able to interact with the platform without writing code.
Benefit from the Next Generation of Analytics
Life sciences organizations transitioning from traditional BI solutions to augmented analytics tool that include these components will see a fast return on their investment. It starts with adoption driven by ease of use and integration into commercial teams’ workflows. For example, organizations that have implemented WhizAI see up to 100% user adoption of our easy-to-use platform.
Additionally, giving business users analytics autonomy with an intuitive platform that allows users to interact conversationally decreases dependence on the company’s data and IT teams by 40-60%. Furthermore, by simplifying processes, the total cost of ownership of data and analytics decreases by as much as 50%.
However, those benefits depend on choosing the right augmented analytics tool for life sciences. The platform should leverage AI, ML, NLP, intelligent automation, and a low-code/no-code platform to meet the analytics needs of commercial teams. Download this whitepaper to learn more or contact us to learn more.