Schools are thinking of ways to keep ChatGPT out. But Life Sciences are rushing to bring WhizAI in!
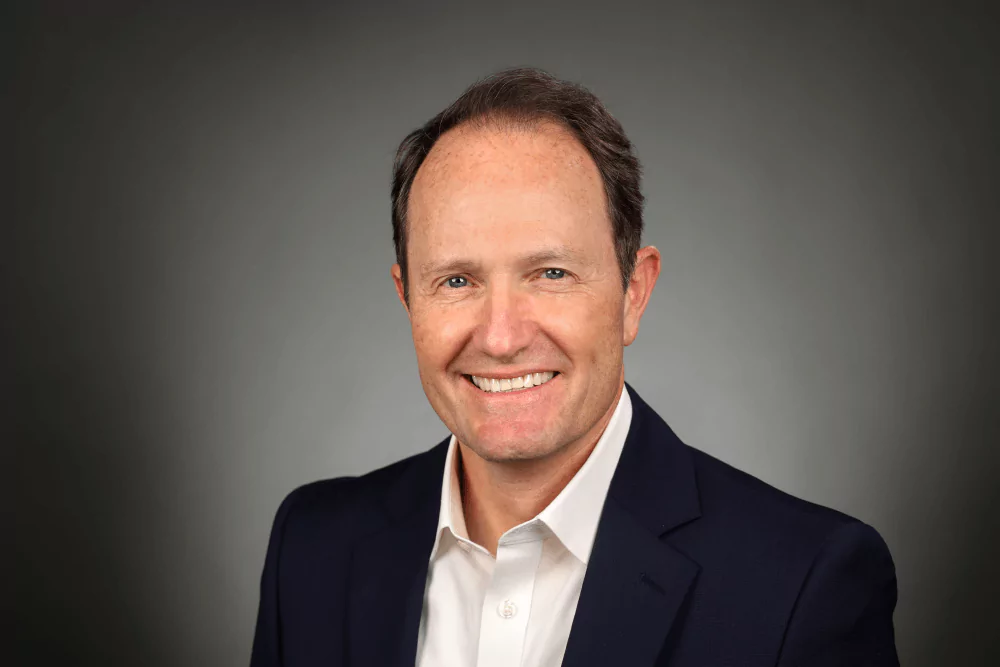

The world is discovering how ChatGPT can answer even complex questions!
- ChatGPT is a powerful AI platform that uses natural language processing (NLP) and large language models (LLMs) to understand and generate human-like responses.
- ChatGPT’s massive training dataset allows it to understand user intent in real time and generate compelling responses.
- LLMs' deep learning algorithms make it possible for users to interact with the platform as naturally as if they were having a conversation with another person.
WhizAI brings more than “ChatGPT experience” to life sciences and healthcare analytics!
ChatGPT has validated the approach WhizAI adopted five years ago
Recognized as a trailblazer by a leading industry analyst, WhizAI introduced conversational AI to life sciences and healthcare analytics. Our platform is providing value to some of the world’s largest Life Sciences companies today.
WhizAI applies the same principles and technologies as ChatGPT -
Natural language understanding (NLU), natural language generation (NLG), and conversational context understanding - to the life sciences domain, WhizAI has built interactive and consumer-oriented user experiences in its analytics solutions.
Both WhizAI and ChatGPT are conversational AI systems
And are similar in providing real-time responses with relevant information based on massive training datasets. But ChatGPT is an Artificial General Intelligence (AGI) system trained on general data on the web and answers all kinds of questions in text form only.
While, WhizAI is a life sciences focused AI platform trained on life sciences data
(patient data, provider data, prescription data, sales data, etc.) and generates actionable insights (in text and visual forms) for life sciences organizations enabling them to make better, faster and smarter decisions at significantly reduced cost.
Today’s analytics systems require expert knowledge of the business domain
To understand the data relationships, perform necessary calculations, infer meaningful insights and generate easy-to-consume visualizations for the users.
While ChatGPT can get away with occasionally wrong responses,
The analytics system must generate consistently accurate data and insights. WhizAI checks all the boxes as the only life sciences-focused analytics platform built for life sciences organizations.
Life Sciences Analytics takes more than ChatGPT
LLM and NLP alone won’t solve the analytics problem for Life Sciences customers.
While ChatGPT elegantly solves for understanding natural language, it doesn’t complete the loop on deep-dive enterprise analytics. They need a purpose-built platform like WhizAI.